Mastering the Art of Bounding Box in Data Annotation
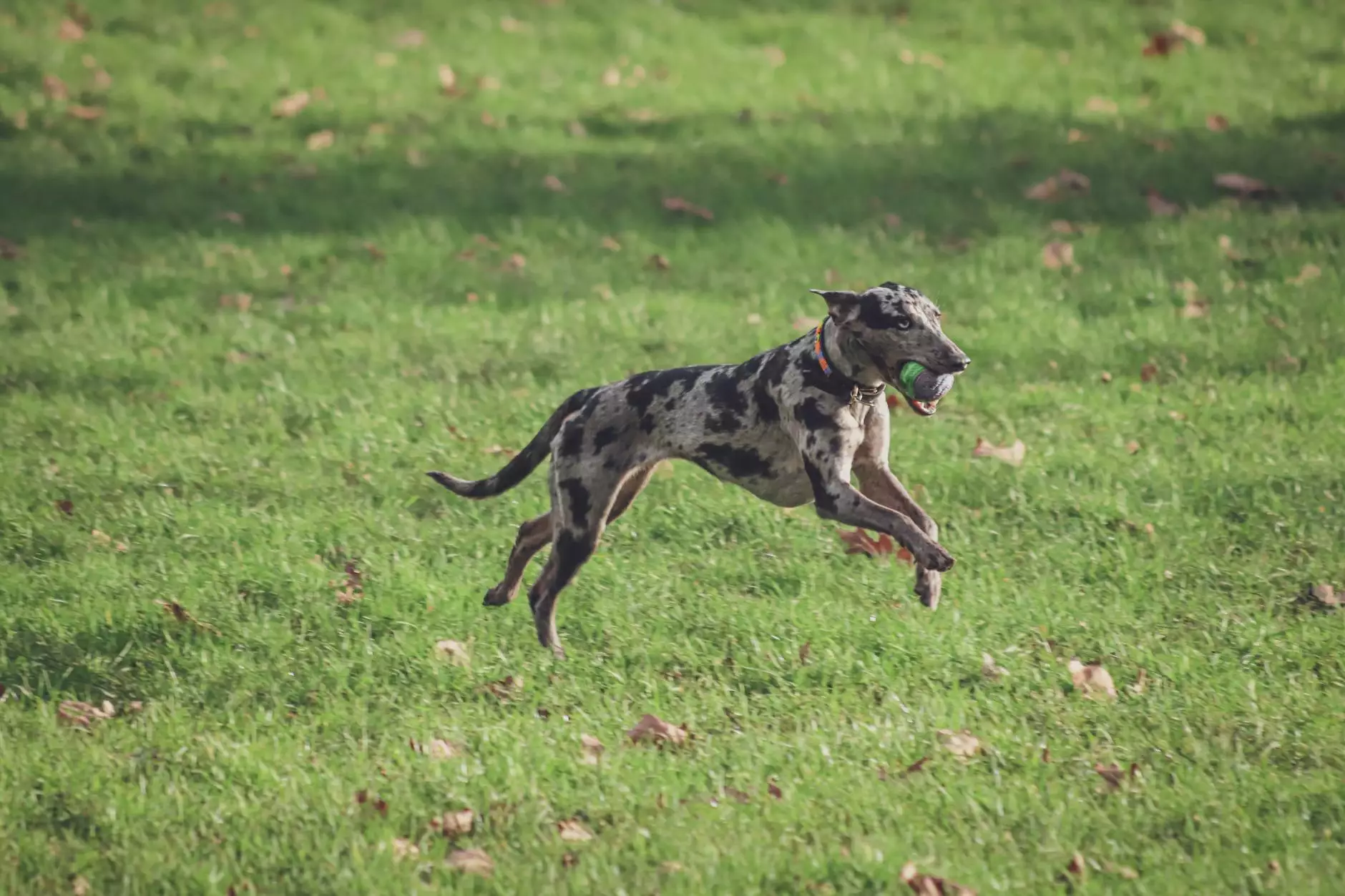
In the rapidly evolving landscape of artificial intelligence and machine learning, the role of data annotation emerges as pivotal. Among the many techniques used for data tagging, the concept of the bounding box stands out, particularly in applications involving image recognition and object detection. This article delves into what bounding boxes are, how they function within data annotation, and why businesses like Keylabs.ai are at the forefront of this technology.
Understanding Bounding Boxes
A bounding box is a rectangular box that encapsulates an object within an image. It is defined by its coordinates—typically the top-left and bottom-right corners—which outline the area of interest. This method of annotation is crucial for training machine learning algorithms, particularly those involved in computer vision tasks.
The bounding box technique is integral to various real-world applications, such as:
- Autonomous vehicles recognizing pedestrians and other vehicles.
- Facial recognition systems identifying faces in different orientations.
- Retail analytics tracking customer interactions with products.
The Importance of Data Annotation Platforms
As businesses harness the power of AI, the need for comprehensive data annotation platforms grows exponentially. These platforms must offer robust tools for effectively implementing bounding boxes to ensure accuracy and efficiency. Keylabs.ai, a leading provider in this space, exemplifies how advanced data annotation tools can transform machine learning capabilities.
Key Features of a Quality Data Annotation Tool
When evaluating data annotation tools, several key features should be on your radar:
- User-Friendly Interface: A simple and intuitive interface allows annotators to work efficiently.
- Precision and Accuracy: The ability to create precise bounding boxes is critical for accurate data training.
- Integration Capabilities: The tool should seamlessly integrate with existing machine learning frameworks.
- Scalability: As data grows, the platform must adapt without compromising performance.
- Collaboration Tools: Built-in features that facilitate teamwork among annotators improve workflow and outcomes.
How Bounding Boxes Enhance Machine Learning
The implementation of bounding boxes significantly enhances machine learning models in the following ways:
1. Improved Object Detection
Bounding boxes enable algorithms to learn how to identify and localize objects within various images. For instance, when a neural network is trained with annotated images, it becomes proficient in distinguishing between different classes of objects, substantially boosting its detection capabilities.
2. Enhanced Model Training
High-quality, well-annotated datasets directly translate to improved model accuracy. When models receive training data with refined bounding boxes, their predictive performance in real-world applications increases significantly.
3. Reduction of False Positives
Bounding boxes help refine the area of interest, which minimizes the chances of false positives in prediction outputs. This precision is crucial in applications like security surveillance, where accurate detections can have significant implications.
Best Practices for Creating Bounding Boxes
To maximize the effectiveness of bounding boxes in data annotation, several best practices should be adhered to:
- Consistency: Ensure that the criteria for creating bounding boxes are consistent across all images.
- Clarity: Each bounding box should distinctly cover the object without excessive padding or tight scrunching.
- Training Annotators: Properly train data annotators on how to create accurate bounding boxes through detailed guidelines and examples.
- Quality Control: Implement rigorous quality control measures to regularly review and validate annotated data.
Business Benefits of Effective Data Annotation
For organizations leveraging AI, the advantages of employing a powerful data annotation platform, like that of Keylabs.ai, are manifold:
1. Accelerated Time to Market
The faster a business can train its models with high-quality annotated data, the quicker it can deploy AI solutions. This expedites the overall project timeline and enhances competitive advantage in the marketplace.
2. Cost Efficiency
Investing in efficient data annotation processes lowers long-term costs. By minimizing errors and the need for rework, businesses can achieve cost savings that positively impact the bottom line.
3. High Return on Investment (ROI)
Ultimately, the goal of data annotation is to create robust machine learning models that deliver value. Effective data annotation, particularly through bounded approaches, enhances model performance, leading to a higher ROI from AI initiatives.
Case Studies: Success Stories with Bounding Boxes
Many organizations have successfully implemented bounding box techniques in their data annotation processes. Here are a couple of notable examples:
1. Autonomous Vehicles
Companies like Tesla train their vehicle detection models with extensive datasets annotated with bounding boxes. This training allows vehicles to accurately detect pedestrians, cyclists, and other obstacles in real-time, significantly enhancing safety and guidance systems.
2. E-commerce and Retail Analytics
Retail giants utilize bounding boxes to track customer interactions with products on their websites and in physical stores. Analyzing customer behavior through annotated images facilitates better inventory management, targeted marketing strategies, and optimized sales processes.
Conclusion: The Future of Bounding Boxes in Data Annotation
In conclusion, the technique of utilizing bounding boxes within data annotation is indispensable for businesses aiming to leverage the power of AI effectively. As platforms like Keylabs.ai continue to innovate and refine their services, the potential for bounding box applications will only grow.
For businesses wanting to remain competitive in the era of intelligent technology, embracing and mastering bounding box techniques in data annotation is not just an option—it’s a necessity. The journey towards effective machine learning starts here, with accurate and detailed data annotation paving the way for groundbreaking advancements in various industries.